OpenEvidence: An AI Solution to Literature Search Woes
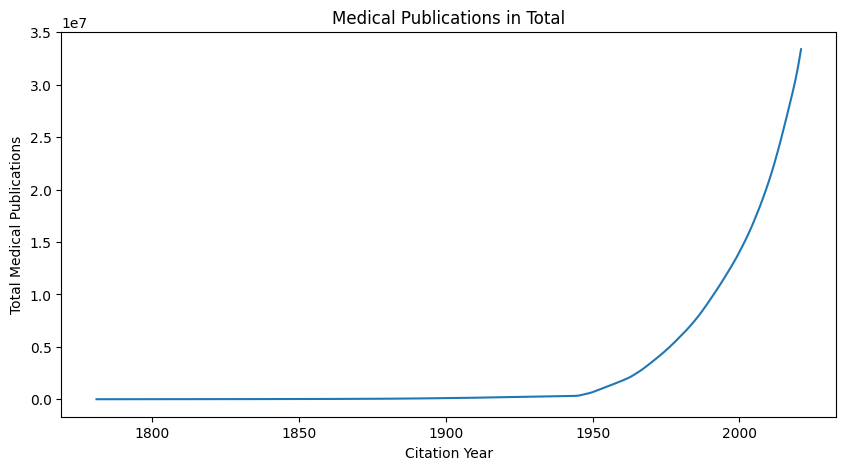
Medicine is a startling juxtaposition of the futuristic and the antiquated.
Right now, a physician somewhere in the country is operating a multi-million dollar surgical robot while sporting a pager from the 1980s.
World class clinicians and researchers are spending hours per day slogging through archaic IT systems built with the forethought and functionality of a barbed wire g-string.
Even the tools we use to browse the medical literature are antiquated, an issue that has become glaringly apparent because of the growing volume of published literature.
Publications are increasing exponentially
PubMed reports 1.6 million medical articles published in the year 2021. In 2010 there were 800,000 (1). This isn't the cumulative number of medical articles; this is the number of articles published in that year alone.
The rate at which we produce medical publications is doubling every 10 years (the Y axis is on a logarithmic scale here):
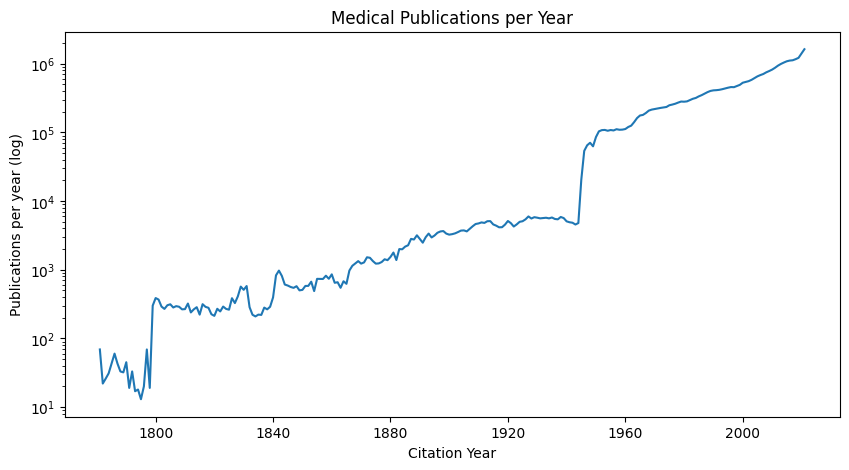
Here is the total number of medical publications on both linear and logarithmic scales:
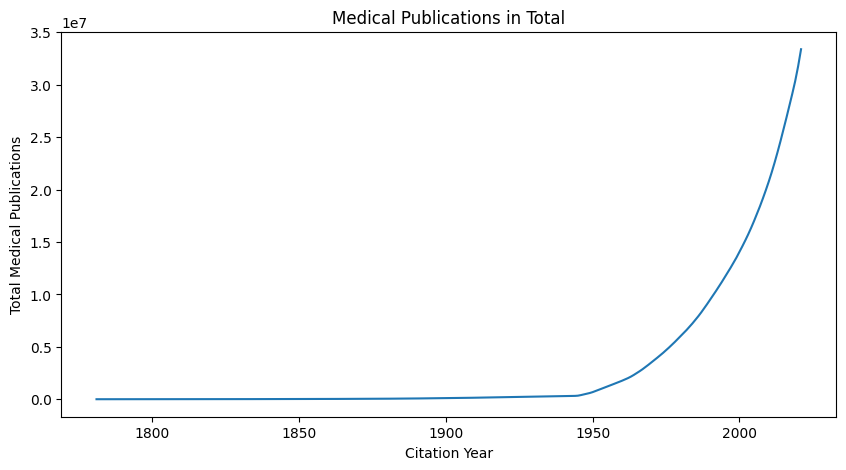
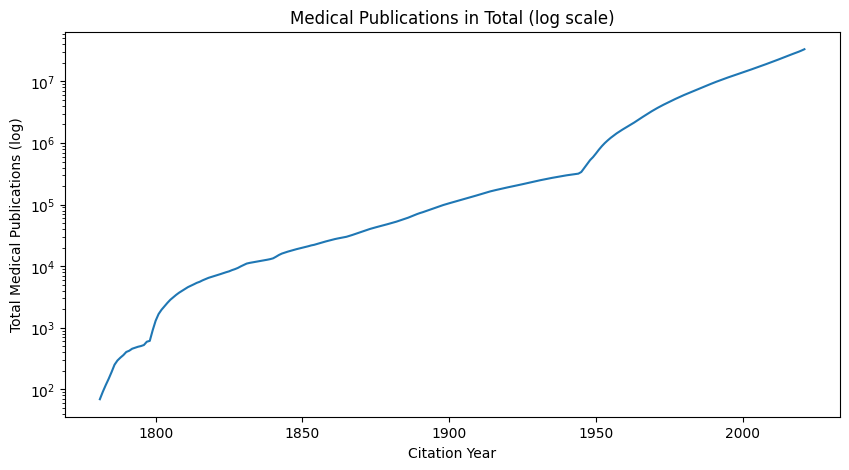
Within the next year, we will surpass 35 million medical publications in the literature. With three articles published each minute on average, reading every piece of medical literature is impossible. Luckily, only a fraction of these publications apply to any individual’s practice.
However, unlike Google, academic literature search tools require meticulously specific wording and syntax.
Searching existing literature is a headache
Here is an example of a literature search string for finding the best surgical procedures for spinal compression fractures:
“Spinal Fracture” [MeSH] OR vertebral compression fracture AND “osteoporosis” [MeSH] AND “Surg*” (2)
Coming up with a query is only the start. You then need to sort through the articles (799 in this example) to determine which are relevant, read them, parse out what they mean, and synthesize this information into an answer to your original question.
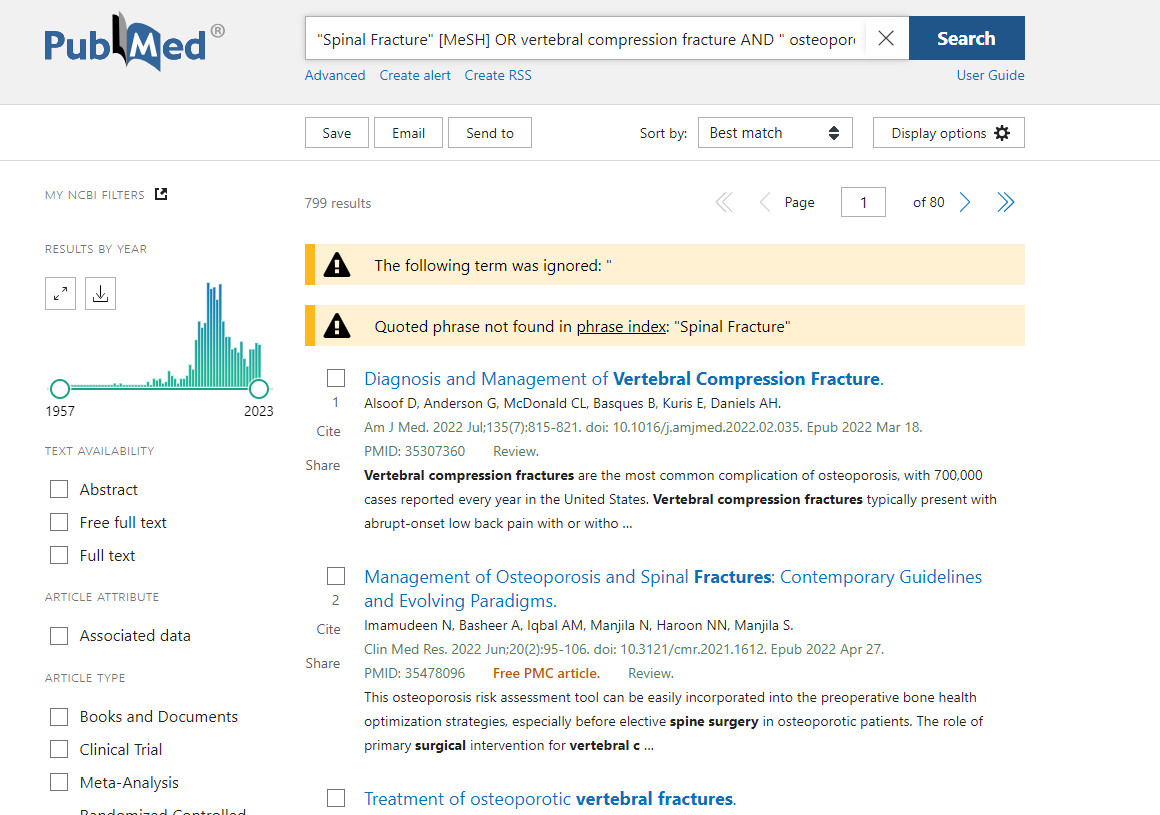
Luckily, technological advancements in AI are offering a solution to this problem.
Natural language processing allows for intuitive search queries. An AI model can then search, summarize, and contextualize relevant literature.
The company OpenEvidence has recently launched a tool to do exactly this for the medical literature. (I am not affiliated with OpenEvidence financially or personally.)
Open Evidence: An AI solution to searching medical literature
In short, OpenEvidence has created a chat bot that takes natural language medical questions and spits out well cited, up-to-date answers.
I asked it, in plain English, the question we were searching for above: “What are the best surgical procedures for spinal compression fractures?”
Here's what it gave me:
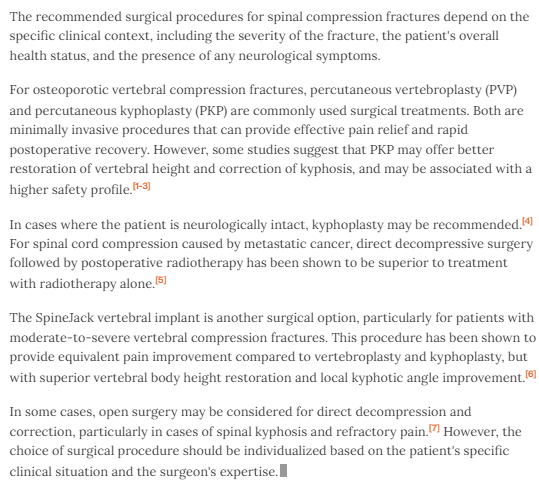
And here are the sources:
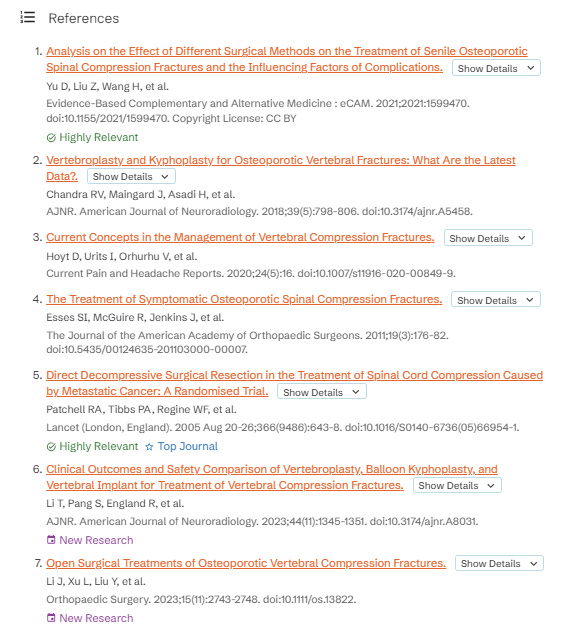
It even recommends follow-up questions to explore the topic further:
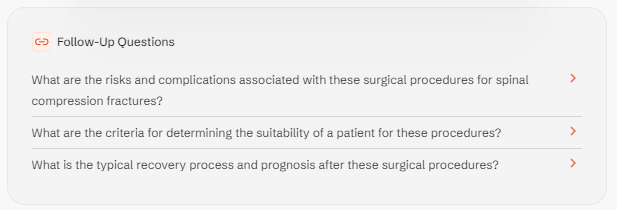
Presence in an academic journal does not guarantee quality. You still need to review the articles to determine if it contains sound research that applies to your practice.
We also do not know the details of OpenEvidence's algorithm and weighting parameters.
Small improvements add up
Literature overload is just one of the thousands of cuts that are contributing to unsustainable rates of burnout among healthcare workers. But the potential of AI solutions reach far beyond assisting with research.
AI tools can ease the burden of many daily tasks for providers across the spectrum of administrative and non-clinical tasks that have piled up over the years, giving us back valuable time.
Let's use this newfound time to get back to the thing that brought us to medicine in the first place: taking care of patients.
Sources: